Jongha Jon Ryu
Postdoctoral Associate at MIT
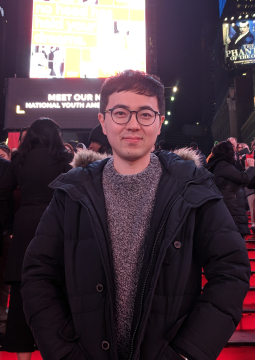
Room 36-677
50 Vassar St
Cambridge, MA 02139
I am currently a postdoctoral associate at MIT, hosted by Gregory W. Wornell. Prior to joining MIT, I received my Ph.D. in Electrical Engineering from UC San Diego, where I was fortunate to be advised by Young-Han Kim and Sanjoy Dasgupta. My graduate study was generously supported by the Kwanjeong Educational Foundation. Before the graduate study, I received my B.S. in Electrical and Computer Engineering and B.S. in Mathematical Sciences (with minor in Physics) with the highest distinction from Seoul National University in 2015.
In general, I aim to develop principled and practical algorithms for machine learning and data science. My recent research topics include:
- Designing algorithms for large-scale problems from first principles
- efficient parametric operator SVD for large-scale problems (NeuralSVD [ICML2024])
- efficient small-k-nearest-neighbors algorithms [TIT2022], [arXiv]
- Learning with efficient & reliable uncertainty quantification
- tight time-uniform confidence sets [TIT2024], [ICML2024]
- identifying pitfalls of evidential deep learning [NeurIPS2024]
- Information-theoretic tools for machine learning and data science
- from universal gambling to time-uniform confidence sets [TIT2024], [ICML2024]
- from universal compression to parameter-free online optimization [AISTATS2022]
- information-theoretic common representation learning (variational Wyner model [arXiv])
- Unifying principles in machine learning
- unified view on density functional estimation with fixed-k-NNs [TIT2022]
- unifying evidential deep learning methods for uncertainty quantification [NeurIPS2024]
- unifying principles for fitting unnormalized distributions via noise-contrastive estimation [arXiv]
As an information theorist by training, I enjoy doing research by simplifying intricate ideas, unifying concepts, and generalizing them to address complex problems.
Check out my resume for more information.
news
Oct 23, 2024 | In this fall, I have given talks on NeuralSVD at MERL, KAIST, KIAS, and Flatiron Institute. |
---|---|
Sep 25, 2024 | Our paper on demystifying the sucess of evidential deep learning methods got accepted at NeurIPS 2024! |
Sep 06, 2024 | I have posted a substantially revised version of the arXiv preprint on minimax optimal learning with fixed-k-nearest neighbors, now including new results on density estimation. |
Aug 21, 2024 | One paper on gambling-based confidence sequences has been accepted at IEEE Transactions on Information Theory! |
Jun 17, 2024 | One paper on new techniques for better score estimation accepted at ICML 2024 Workshop on Structured Probabilistic Inference & Generative Modeling. |
Selected publications
- ICMLOperator SVD with Neural Networks via Nested Low-Rank ApproximationIn Proc. Int. Conf. Mach. Learn. (ICML) , July 2024
- arXivLearning with Succinct Common Representation with Wyner’s Common InformationJuly 2022Submitted. A preliminary version of this manuscript was presented at the Bayesian Deep Learning Workshop at NeurIPS 2018, and an abridged version of the current manuscript was presented at the Bayesian Deep Learning workshop at NeurIPS 2021.